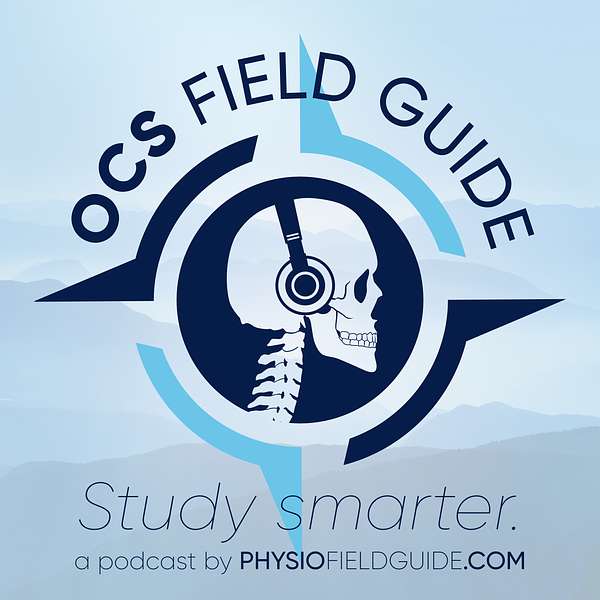
OCS Field Guide: A PT Podcast
Pass the OCS exam by studying smarter, not harder. This podcast is for physical therapists looking to become board-certified specialists in orthopedics. Use code FIELDGUIDE for $101 off a MedBridge subscription.
OCS Field Guide: A PT Podcast
Research Part I: Basics and Statistics to Memorize
A large number of exam takers who fail to pass the OCS exam report that the section on research was one of their lowest-scoring sections. Dr. David Smelser discusses research basics as well as statistics and important values you will need to memorize to ace the research portion of the exam. In this episode, David covers levels of evidence, types of variables, p-values and alpha levels, type I and type II errors, effect sizes, Cohen's kappa, and likelihood ratios. And just to keep things interesting, these values are reinforced with real data from studies that could be relevant for the exam.
Use code FIELDGUIDE for 40% off a MedBridge subscription.
Find more resources and subscribe to practice questions at PhysioFieldGuide.com.
Use code FIELDGUIDE for 40% off a MedBridge subscription.
Support the podcast and get study guides and bonus episodes at Patreon.com/physiofieldguide.
Find more resources and subscribe to practice questions at PhysioFieldGuide.com.
In this episode, we’re going to discuss research and statistics. Now, I know what you’re thinking. Why would we start the podcast off with the most exciting topic possible? Aren’t we going to run out of fun and exciting material if we’re already doing research and statistics in the first full episode? Don’t worry; there is plenty of fun to come.
In reality, we’re starting with research and statistics because this is one area that a lot of prep courses and material neglect, and a lot of therapists who didn’t pass their OCS exam last complained that this area was one of their lowest scoring sections. Last year, the average score in the section called “Critical Inquiry for Evidence-Based Practice” was a 71, which made it the second lowest-scoring section of the OCS exam. Remember that a passing overall score was around a 70%, so about half of exam takers were scoring below passing on this section of the exam.
In other words: this is an important section where a lot of people struggle. So we’re going to break it down for you up front.
I’m going to cover the most important material in two episodes. In this episode we’re going to cover some research basics, and then we will talk about some statistics and values that you simply must memorize to pass the OCS exam. If you feel confident in your grasp of research basics, you can skip to skip forward to minute seven to get to the more intermediate material and the values you need to know. Next episode, we’re going to talk about some psychological principles of research that might pop up as exam questions—and that most research classes in PT school don’t spend much time discussing.
First, the basics.
If the board is going to label you a specialist, they want to see that you will be able to read, interpret, and apply research correctly. The first part of that process is distinguishing between good and bad research. So the OCS exam is going to do its best to make sure you understand the difference between good evidence and bad evidence.
Not all evidence is created equal. The Clinical Practice Guidelines contain summaries of how individual articles are ranked in terms of strength of evidence. The levels are designated with Roman numerals, starting with the highest strength of evidence, as follows:
- Evidence obtained from high-quality diagnostic studies, prospective studies, or randomized controlled trials.
- Evidence obtained from lesser-quality diagnostic studies, prospective studies, or randomized controlled trials (e.g., weaker diagnostic criteria and reference standards, improper randomization, no blinding, <80% follow-up).
- Case controlled studies or retrospective studies
- Case series
- Expert opinion
It is possible that you will be given some examples of research studies and asked to identify the highest or lowest quality study. For the most part, it’s easy to recognize that a high quality randomized controlled trial falls into the highest tier of research. What might be a little trickier is to remember that a high quality retrospective study is level III evidence, which is lower than a lesser quality prospective study, which is level II evidence. It also might be tricky to remember that expert opinion is the lowest level, which means a case series is considered higher quality evidence than an expert opinion paper.
Let’s cover types of variables. Generally in an experimental design, researchers are tracking two types of variables: dependent variables and independent variables. You could be given a research design and asked to identify what variable is which. So remember that the independent variable is what the researchers decide to manipulate, and the dependent variable is the outcome. The outcome depends on the effectiveness of the independent variable. So if researchers are comparing the effectiveness of manual therapy to the effectiveness of ultrasound at reducing a patient’s pain, the independent variable is the treatment type (manual therapy or ultrasound), and the dependent variable is the patient’s pain level. The pain level depends on the effectiveness of the treatments.
Let’s talk p-values. In a research design like this, where researchers are comparing two or more groups, they are going to use p-values. Basically, a p-value tells you the probability that the differences you are seeing between the groups occurred due to chance. So in the example we just mentioned, if ultrasound reduced pain by 4 points on the numeric pain rating scale and manual therapy reduced pain by 5 points, we need to know if the difference between treatments is due to chance or if manual therapy is really more effective than ultrasound. Let’s say we run our analysis and find that the p-value is 0.09. This basically means there is a 9% chance the difference between groups is due to chance.
So researchers have to decide how certain they want to be that their results are not due to chance. They almost always settle on 95% certainty, which means any p-value lower than 0.05 is considered “statistically significant.” The point at which the researchers decide their results are statistically significant is called the alpha level. So if the alpha level is 0.05, any p-value lower than 0.05 is considered statistically significant. If the researchers decide to set the alpha level at 0.03, then the p-value would have to be lower than 0.03 to be considered statistically significant.
Now if I was a mean OCS test item writer, I could propose a research scenario where the alpha level was set to 0.03, give you a p-value of 0.04, and ask you if the results are statistically significant. The answer would be no, because the p-value was not lower than the alpha level.
But hopefully you’re familiar enough with research that the last few minutes have just been review. So let’s get into some intermediate concepts.
Type I and type II errors. These are concepts that usually make sense when you read about them, but by the third hour of the OCS exam, the two errors start to blend together.
We just discussed the p-values, or the probability that the results we obtained were due to chance. Even with a very low p-value, there’s still a chance the results were a fluke (or the result of bad research methods). In this case, researchers might conclude there is a significant difference between groups when—in reality—there isn’t. This is called a type I error. This is sometimes referred to as “backing a loser.”
A type II error occurs if the researchers do not find a statistically significant difference between their groups, when—in reality—one group is different from the other. This is sometimes called “missing a winner,” and it’s is often due to having too few subjects in the study. Since there is not enough data, researchers were unable to detect a difference even though a difference exists. So If you’re asked a question on the exam about how to decrease the chances of committing a type II error, you want to increase the number of subjects in the study.
I mentioned the terms “backing a loser” and “missing a winner.” These phrases will help you remember which error is which. Imagine a scoreboard where one team has one point and the other team has two points. If you picked the team with one point, you backed the loser. In research terms, your paper claimed an intervention was effective when—in reality—it is not. It is a loser. It has the lower score—one point. You committed a type I error.
In contrast, if you failed to pick the team with two points, you “missed a winner.” In research terms, you said an intervention is not effective when—in reality—it is. You “missed a winner,” you failed to pick the team with two points—you committed a type II error. So in the middle of the exam, when you have to remember which error is which, imagine your scoreboard and remember the phrases, “backing a loser” (that’s a type I error) and “missing a winner” (a type II error).
Now we’re going to get into some values that you’re going to have to memorize. If I was studying for this exam again, I would bookmark this portion of the podcast and listen to it over and over to help myself remember these values.
Effect sizes. In the imaginary research example we’ve been using, let’s pretend the researchers found a statistically significant difference between manual therapy and ultrasound. Let’s say they found that manual therapy was better at reducing pain. A clinical specialist’s next question would be: how much better? That’s what the effect size tells us.
It is very likely that you will have to interpret an effect size on this exam. The question might describe a research scenario and then inform you that the effect size was found to be 0.6. How large or small of an effect is that?
Here are the effect size values you need to remember:
0.8 and up is a large effect size.
0.5 up to 0.7999 is a moderate effect size.
0.2 up to 0.4999 is a small effect size.
Anything below 0.2 is a trivial effect size.
So your cut-offs are 0.8 and up for large, at least 0.5 for moderate, at least 0.2 for small, and anything lower is trivial. I’m going to say that one more time. Your cut-offs are 0.8 and up for large, at least 0.5 for moderate, at least 0.2 for small, and anything lower is trivial.
So in the example above, if the research found an effect size of 0.6, then we could say the effect size for manual therapy on pain is moderate.
Let’s move on to test reliability. When we look at a test or measure, we want to know that we are going to get approximately the same result each time we take it. Interrater reliability means that several different clinicians will all get the same result. Intrarater reliability means that the same clinician will get the same result when performing the test multiple times on the same person. Remember, the internet connects multiple listeners to the same podcast, so interrater reliability involves multiple clinicians performing the same test.
For the OCS exam, you will need to know how to interpret reliability values. The most common measure of reliability is Cohen’s kappa. Cohen’s kappa, which looks like a small upper-case K, runs on a scale from 0 to 1. Zero represents absolutely no reliability—that you might as well just flip a coin—and one represents perfect reliability—that the result is the same when performed on the same individual every time. Now the cutoff values for Cohen’s kappa vary a little bit depending on your source—you might say they’re unreliable—but these are generally good cutoffs to remember:
0 means no better than chance.
<0.4 indicates poor reliability.
0.4 to 0.6 indicates fair reliability.
0.6 to 0.75 indicates good reliability.
>0.75 indicates excellent reliability.
1 is perfect reliability.
So again, the cutoffs are: less than 0.4 for poor, 0.4 to 0.6 for fair, 0.6 to 0.75 for good, and greater than 0.75 for excellent.
Let’s use a real-world example. A study in 1990 by Mior, McGregor, and Schut examined interrater reliability of sacroiliac motion palpation tests for sacroiliac dysfunction. So they were looking at special tests like Gillet’s test (also called the Stork test) or the standing forward flexion test. They examined chiropractic students in their final year of school before and after 1 year of clinical experience, and they also examined more experienced chiropractors. They found that the interrater reliability for chiropractic students ranged from a kappa coefficient of 0 to 0.3. So how reliable is sacroiliac motion palpation testing for chiropractic students? Where do these values fall? Remember that less than 0.4 is poor reliability, and zero is no better than chance. So the reliability for chiropractic students was poor to no better than chance.
Now when researchers examined the experienced chiropractors, they found kappa values of 0 to 0.167. How does that compare? That’s even worse—but still poor to no better than chance.
(And can I just take a second to point out that this has some serious implications for all of us in the clinic doing motion palpation testing of the SI joint?)
Anyway, moving on to our last list of numbers: likelihood ratios. So we’ve talked about effect sizes, which are usually used in research that examines interventions. And we’ve talked about reliability, which is looking at how effectively clinicians can get consistent results from a measure. Likelihood ratios tell you what to do with positive or negative test results. A positive likelihood ratio tells you how much you should increase your suspicion of a certain condition based on a positive test result. A negative likelihood ratio tells you how much you should decrease your suspicion of a condition based on a negative test result. Positive likelihood ratios are going to be larger than one; negative likelihood ratios are going to be smaller than 1. Here are my simplified cutoff values:
For positive likelihood ratios,
Anything >10 indicates a large shift in probability towards a diagnosis.
Anything 5-10 indicates a moderate shift in probability.
Anything less than 5 indicates a small shift in probability, all the way down to no change at 1.
For negative likelihood ratios,
Anything <0.1 indicates a large shift in probability away from a diagnosis.
Anything from 0.1-0.2 indicates a moderate shift in probability.
Anything above 0.2 indicates a small shift in probability, with a value of 1 indicating no change.
Again, for positive: >10 is large. 5-10 is moderate. Less than 5 is small. For negative, less than 0.1 is large. 0.1-0.2 is moderate. Larger than 0.2 is small.
So, as an example, Laslett’s SI joint pain Clinical Prediction Rule has a positive likelihood ratio of 4.16. How much should our suspicion shift toward SI joint pain if the cluster is positive? 4.16 is a small shift in probability (although it’s close to the moderate cutoff). The same clinical prediction rule has a negative likelihood ratio of 0.12. So if the cluster is negative, how should our suspicion against SI joint pain be affected? 0.12 is a moderate negative likelihood ratio (getting close to the 0.1 cutoff for a large value). So Is Laslett’s cluster better for ruling in SI joint pain or ruling it out? Based on these likelihood ratios, it is better at ruling out.
That wraps up my discussion of research-related values that you have to memorize for the OCS exam. Next episode I’m going to leave the strings of numbers behind and talk about some psychological features of human research that we, as PTs, don’t usually talk about, but that could show up as OCS exam questions.